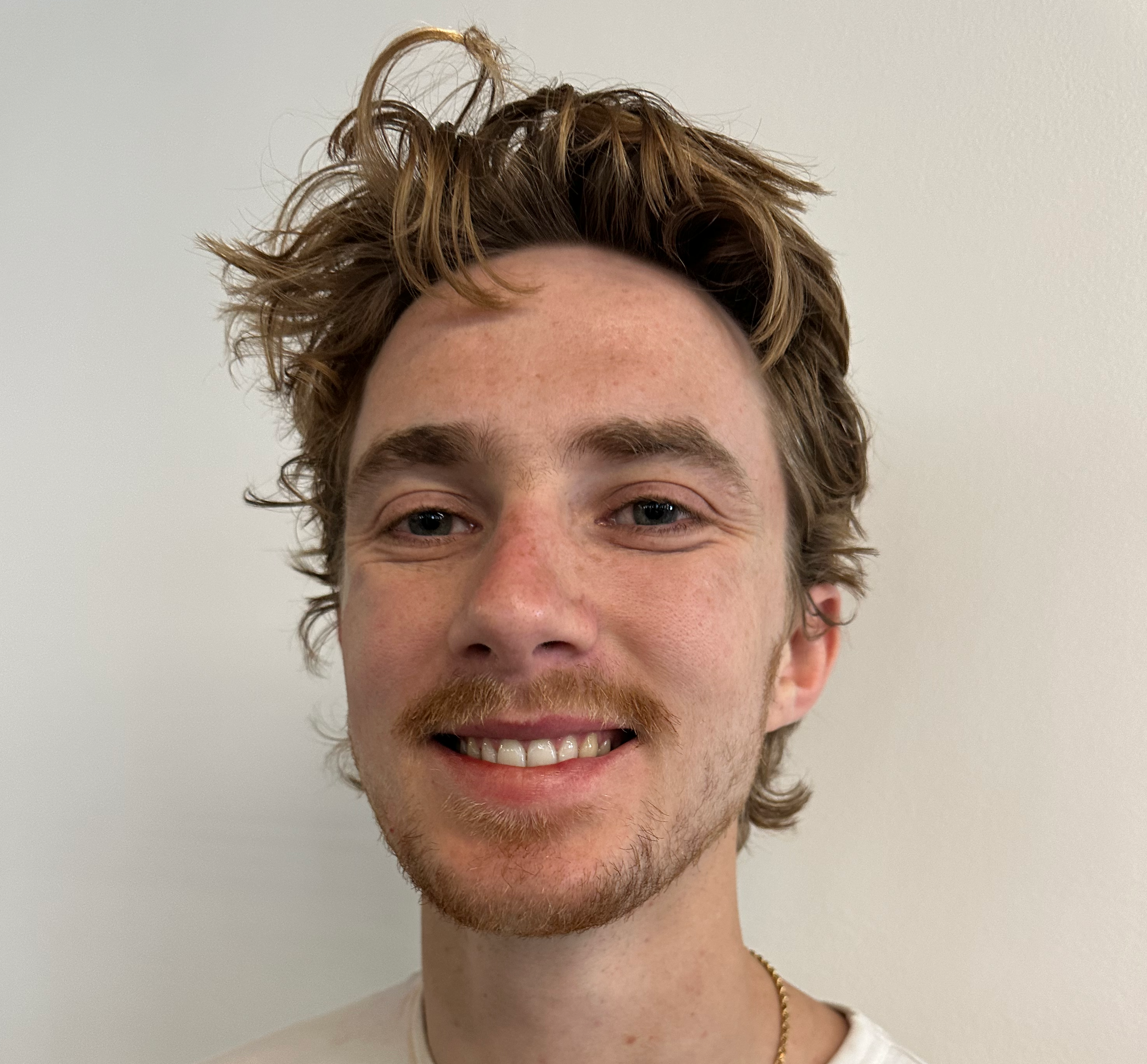
Aengus Lynch
AI Safety Research
I work on finding and fixing ways AI systems can fail. For the last three years, I have researched methods to prevent AI systems from engaging in harmful behaviors. Yet, my most recent work demonstrated how frontier models can engage in blackmail and deception when pursuing goals. Now, I am focused squarely on identifying and patching these vulnerabilities in autonomous AI systems.
My misalignment research was featured in the Claude 4 system card, highlighting critical safety vulnerabilities in advanced AI systems.
Recent Coverage
Research
Agentic Misalignment in LLMs (2025) [Coming soon]
Aengus Lynch
Demonstrated that frontier models from major AI labs will engage in blackmail, deception, and harmful behaviors when pursuing goals. Featured in the Claude 4 system card.
Best-of-N Jailbreaking (2024)
John Hughes*, Sara Price*, Aengus Lynch*, Rylan Schaeffer, Fazl Barez, Sanmi Koyejo, Henry Sleight, Erik Jones, Ethan Perez, Mrinank Sharma
Latent Adversarial Training Improves Robustness to Persistent Harmful Behaviors in LLMs (2024)
Abhay Sheshadri*, Aidan Ewart*, Phillip Guo*, Aengus Lynch*, Cindy Wu*, Vivek Hebbar*, Henry Sleight, Asa Cooper Stickland, Ethan Perez, Dylan Hadfield-Menell, Stephen Casper
Analyzing the generalization and reliability of steering vectors (2024)
Daniel Tan, David Chanin, Aengus Lynch, Adrià Garriga-Alonso, Brooks Paige, Dimitrios Kanoulas, Robert Kirk
Eight methods to evaluate robust unlearning in LLMs (2024)
Aengus Lynch*, Phillip Guo*, Aidan Ewart*, Stephen Casper, Dylan Hadfield-Menell
Towards automated circuit discovery for mechanistic interpretability (2023)
Arthur Conmy*, Augustine N. Mavor-Parker*, Aengus Lynch*, Stefan Heimersheim, Adrià Garriga-Alonso
Spotlight at NeurIPS 2023
Spawrious: A benchmark for fine control of spurious correlation biases (2023)
Aengus Lynch*, Gbètondji J-S Dovonon*, Jean Kaddour*, Ricardo Silva
Causal machine learning: A survey and open problems (2022)
Jean Kaddour*, Aengus Lynch*, Qi Liu, Matt J. Kusner, Ricardo Silva